How big data analytics can accelerate a project?
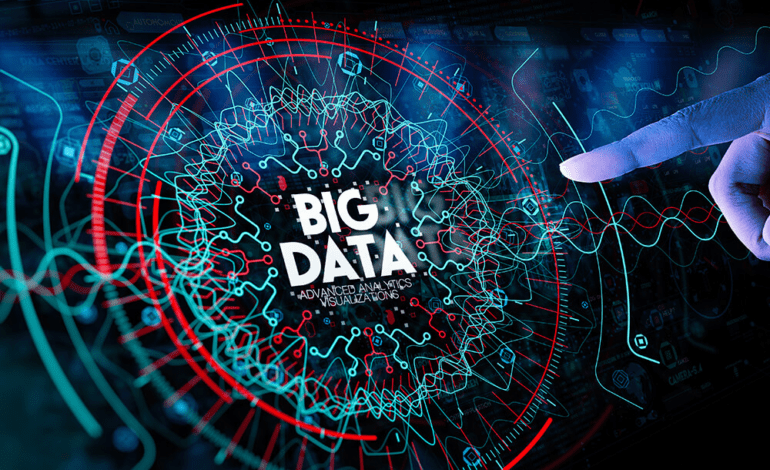
To draw an analogy with metal mining, the unprocessed ore must be extracted from the ground, transported to factories that use mechanical and chemical processes to obtain the metal, and only then can it be used in jewelry or other products.
Likewise, data flows from the raw form to the level of business understanding.
Collection: Business-oriented big data is typically sourced from machine or IoT data (such as data streams, server logs, and RFID logs), transaction data (such as website activity, point-of-sale data from physical storage), and cloud data (such as stock exchange stock prices, social media channels). This data is often unstructured (lines of text or images) or semi-structured (log data with time stamp, IP address, and other information). In the general definition of big data, such data is large (from terabytes to petabytes), high growth rates (many terabytes of new data per day) and a high level of diversity (hundreds of different types of servers and applications, each of which creates information in its own formats).
Clarification: Quite often, organizations also use the EDW data warehouse, which serves as a central repository for structured data that needs analysis. EDWs are not only designed for storage, but also have robust ETL (extract, transform, load) capabilities, so they play an additional role with Hadoop clusters. EDWs can pull data directly from a data source, SAN (storage area network) or NAS (network attached storage), or Hadoop clusters. Since the data in EDW is structured and not raw, it is easier to query and present a higher level of value than the original data.
Analysis: The typical business user needs the flexibility to retrieve data from multiple sources and should be shielded from the details of where the data comes from or how it is organized. Data modeling should be fast and easily cover different data sources. This environment (environment) not only reduces the burden on IT to meet business requirements, but also enables business users to include additional data in their analysis in a timely manner.
Business users are constantly striving to make it more efficient to access, filter, and analyze data – and gain insight – without using data analysis solutions, this requires specialized skills. They need better, simpler ways to navigate massive amounts of data to find what works for them and get their specific important questions answered so they can make faster decisions. In this case, you can try reverse ETL explained by Meltano team. In a nutshell, ETL is data pipeline that ensures that data is transformed into compatible formats before being loaded into data warehouses.
It is important to understand that:
• The most important data may not be present in the Big Data repository. Often, data from a BigData store acts as ancillary data. For example, a spreadsheet or small database containing customer satisfaction survey results can be the basis for an analytic query, and Big Data data allows a user to correlate a customer service, customer, or support history with a satisfaction score.
• The data required for analysis can be scattered across multiple repositories. The process of creating an enterprise data warehouse can not only involve copying data from an operational data source, but it can also involve modeling and transforming metadata. Since this can be time consuming or costly, some of the operational sources may remain separate. They do not require the cost and effort of loading into the data warehouse.
Two important aspects to consider when working with big data are determining the need (relevance) and context of the information.
Necessity: the right (Right) information for the right person at the right time. Аpproach has always been to understand what business users are demanding from their analysis, not to force a solution that might not be acceptable. Having access to the right data at the right time is more valuable to users than having access to all the data all the time. For example, branch managers of banks may want to understand (receive) sales, customer information, and market dynamics in their branches rather than the entire nationwide branch network. With this simple approach, there is a transition from one large amount of data to one of the necessary ones. Big data development company.